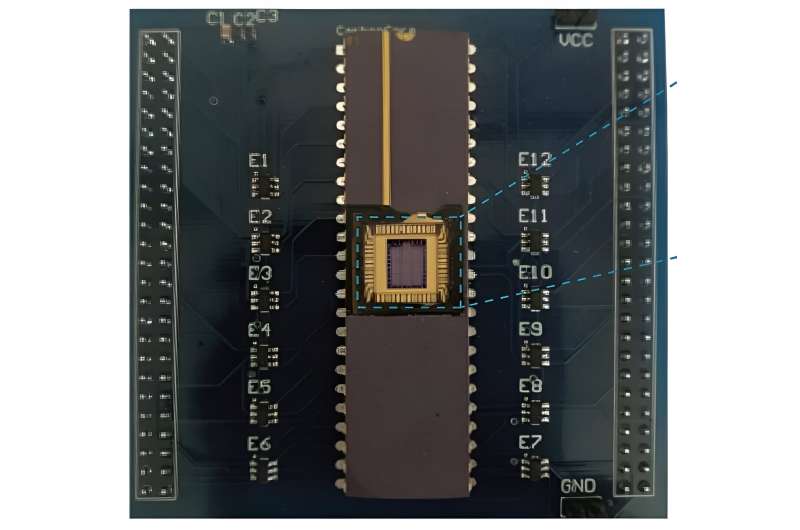
Artificial intelligence (AI) and machine learning tools have proved to be highly effective in tackling various tasks that entail analyzing data and making accurate predictions. Despite their advantages, these tools have significant computational demands, and when running on existing processing units, they can consume a lot of energy.
Researchers at Peking University and other institutes in China recently developed a highly promising tensor processing unit (TPU) based on carbon nanotubes that could be used to run AI algorithms more energy-efficiently. This carbon nanotube-based tensor processing chip, presented in a paper in Nature Electronics, could be a key breakthrough on the path towards developing next-generation chips.
“We successfully developed the world’s first tensor processor chip (TPU) based on carbon nanotubes,” Zhiyong Zhang, co-author of the paper, told Tech Xplore. “We were inspired by the fast development of AI applications as well as TPU by Google. From ChatGPT to Sora, artificial intelligence is ushering in a new revolution, but traditional silicon-based semiconductor technology is increasingly unable to meet the processing needs of massive amounts of data. We have found a solution in the face of this global challenge.”
In computer science, systolic arrays are networks of processors that rhythmically compute data and allow it to pass through them freely, similar to how blood flows through the human body. Zhang and his colleagues developed a new efficient systolic array architecture using carbon nanotube transistors, field effect transistors (FETs) with channels made of carbon nanotubes instead of conventional semiconductors. Building on this new architecture they developed, they created the world’s first carbon-nanotube-based TPU reported to date.
“The chip is composed of 3,000 carbon nanotube field-effect transistors, organized as 3*3 processing units (PEs),” Zhang explained. “These 9 PEs form a systolic array architecture, which can perform two-bit integer convolution and matrix multiplication operations in parallel.”
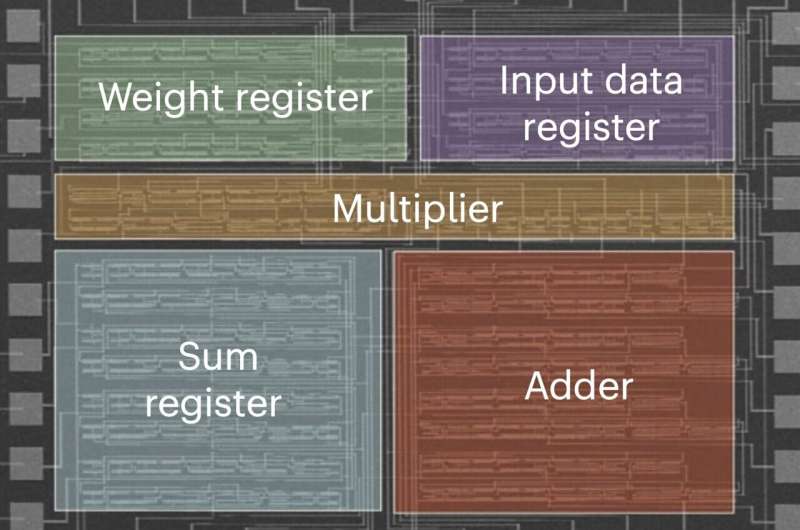
The tightly coupled architecture introduced by Zhang and his colleagues supports the flow of systolic input data. This flow of data through the architecture reduces read and write operations of static random-access memory (SRAM) components, which translates into significant energy savings.
“Each PE receives the data from its upstream neighbors (up and left), independently calculates a partial result within itself, and passes it downstream (right and down),” Zhang said. “Each PE is designed for 2-bit MACs and matrix multiplication on signed and unsigned integers. Combined with systolic data flow, the CNT TPU could accelerate convolution operations in NN applications.”
The team’s proposed system architecture was carefully designed to accelerate tensor operations performed by artificial neural networks, easily switching between integer convolutions and matrix multiplications. The tensor processing chip they developed based on this architecture could be a crucial milestone for the development of new, high-performing integrated circuits based on low-dimensional electronics.
More information:
Jia Si et al, A carbon-nanotube-based tensor processing unit, Nature Electronics (2024). DOI: 10.1038/s41928-024-01211-2.
© 2024 Science X Network
Citation:
The first tensor processor chip based on carbon nanotubes could lead to energy-efficient AI processing (2024, August 21)
retrieved 21 August 2024
from https://techxplore.com/news/2024-08-tensor-processor-chip-based-carbon.html
This document is subject to copyright. Apart from any fair dealing for the purpose of private study or research, no
part may be reproduced without the written permission. The content is provided for information purposes only.