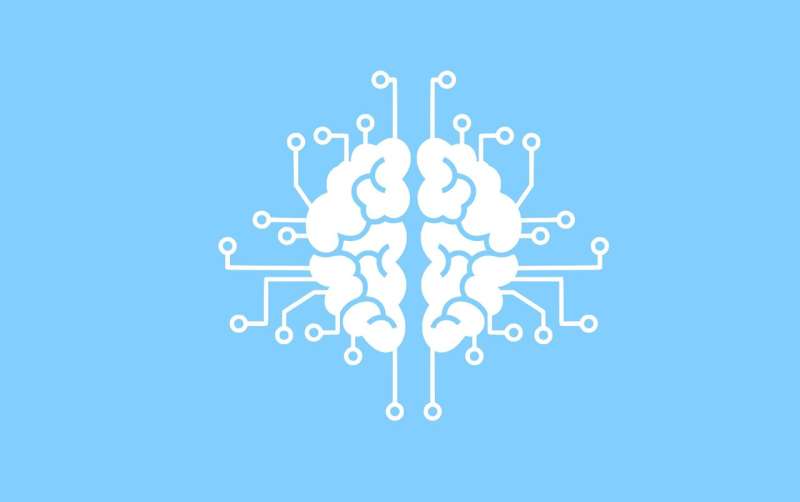
Recent algorithms in artificial intelligence (AI) are often referred to as “black box” models, meaning their inputs and operations are not visible to the user or any other party, making their decisions difficult to interpret. eXplainable AI (XAI) is a class of methods that seeks to address the lack of AI interpretability and trust by explaining AI decisions to customers.
Many experts believe that regulating AI by mandating fully transparent XAI leads to greater social welfare. However, a new study from the Tepper School of Business at Carnegie Mellon University challenges this notion, finding that such regulation may lead to less-than-optimal outcomes for both companies using AI and consumers.
The study conducted by researchers at Carnegie Mellon and the University of Southern California has been accepted for publication in Marketing Science.
“Consumer advocates are increasingly calling for regulating AI and mandating increasingly transparent XAI,” said Behnam Mohammadi, Ph.D. candidate at the Tepper School, who coauthored the study.
“Companies are facing pressure from legislators and customers to adhere to accountable AI practices, but we know little about the economic implications of XAI. We took a deep dive into the complexities of XAI regulations to learn about their impact on competition among companies and social welfare.”
In recent years, the use of AI models for decision-making has significantly increased, with billions of dollars spent globally on this technology. But a key challenge is the interpretability of AI’s decisions and predictions.
Early models were easily interpretable, but the latest methods (e.g., deep neural networks) feature opaque decision systems, and many people are reluctant to adopt algorithms that are not easily interpretable, controllable, and trustworthy. This is especially problematic when black box models result in biased outcomes, such as showing fewer ads for high-paying jobs to women than to men or failing to recognize non-White faces.
XAI is a class of methods that aims to produce “glass box” models that are explainable to people while retaining prediction accuracy. They seek to enable people—including non-technical experts—to understand, trust, and effectively manage emerging AI systems. Along with calls by consumer activists for this type of method, XAI has been gaining traction across health care, retail, media and entertainment, and aerospace and defense industries
Do customers and companies want different things from XAI? And if so, how should the rules for XAI be set? The researchers studied a situation where two big companies dominate the market, specifically focusing on the insurance industry—a field that uses AI to decide rates. The study found that in places with no rules, companies and customers often want different levels of explanation from AI.
“Sometimes, full explanations can be bad for customers. They might prefer that companies don’t explain everything, which could lead to better products,” said Nikhil Malik, assistant professor of marketing at the Marshall School of Business at the University of Southern California, who coauthored the study.
The study concluded that partial explanations might be better for both consumers and companies. In fact, the authors were surprised to find that full explanations may make consumers worse off; consumers may prefer that one or both firms set less than full XAI, which in turn would encourage the firms to offer higher-quality products.
More information:
Behnam Mohammadi et al, Regulating Explainable AI (XAI) May Harm Consumers. Marketing Science (2024). papers.ssrn.com/sol3/papers.cf … ?abstract_id=4602571
Tepper School of Business, Carnegie Mellon University
Citation:
Study finds limited explanations in AI might benefit consumers (2024, June 18)
retrieved 18 June 2024
from https://techxplore.com/news/2024-06-limited-explanations-ai-benefit-consumers.html
This document is subject to copyright. Apart from any fair dealing for the purpose of private study or research, no
part may be reproduced without the written permission. The content is provided for information purposes only.