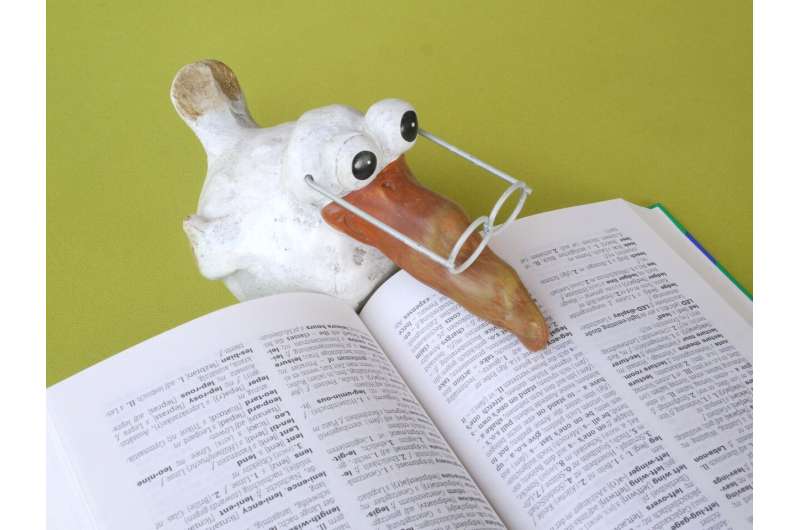
ChatGPT and its ability to hold conversations and produce written content have been the focus of a lot of attention in the last year in the field of technology and artificial intelligence. However, AI has been around for some time, helping us in all sorts of everyday tasks, from navigation systems to social network algorithms, not to mention machine translation. Ever since neural machine translation (NMT) systems began to be used on a widespread basis a few years ago, AI has seen exponential growth in its uptake in the translation industry. This has led to new challenges in the relationship between human and machine translators.
Today, the post-editing of machine translations is the second-most sought-after skill among language service providers and is the task with the greatest growth potential, according to the European Language Industry Survey. Translators edit unprocessed machine translations, correcting texts produced by artificial intelligence. This brings with it many advantages for human translators, but also significant problems if the quality of the machine translation is poor. This is why the ability to objectively assess the quality of machine translation tools is essential for the sector.
Two researchers from the Universitat Oberta de Catalunya (UOC), Antoni Oliver, member of the Interinstitutional Research Group in Linguistic Applications (GRIAL-UOC), coordinator of the TAN-IBE project and member of the UOC’s Faculty of Arts and Humanities, and Sergi Álvarez-Vidal, a fellow GRIAL-UOC researcher, have developed a new method for assessing work by AI to improve translators’ work, boosting their capabilities with the potential of machine translation, and enhancing the quality of the end result for all users.
A new method for assessing AI in translation
Most translation and language services companies analyze the quality of AI tools in a similar way, using automated metrics. In their latest study, Oliver and Álvarez-Vidal have analyzed the degree to which these automated assessment systems actually help to choose tools that really facilitate the subsequent work of human translators. To do this, they measured the so-called post-editing effort, calculating the time, the breaks taken and the keys used by translators to gain an in-depth understanding of the difficulties involved in editing and correcting a text produced by machine translation.
The paper is published in the journal Ampersand.
“We have concluded that there is no direct relationship between what automated quality assessment metrics say and the actual post-editing effort involved,” said Oliver. “We therefore felt that there was a need to add a further step to the quality assessment system.” So it is that the researchers suggest complementing automated assessment systems with another program that helps evaluate the actual effort put into post-editing. This will allow companies to choose an AI tool that actually increases the efficiency of the translation process.
“We have added a further step: translators translate a sample of the machine translation with a special program we have developed. This program allows us to gather a range of data and decide whether the effort made by the translators is less than that with other systems,” explained Álvarez-Vidal. “If it is less, it means that this machine translation tool works for the translation company’s workflow.”
AI, support for human translators
Machine translation systems are widely used in the translation industry, although the end results are always reviewed by people. During this post-editing work, human professionals accept, amend and correct or even reject in its entirety the result produced by machines.
“In this regard, it is extremely important to think about who is at the heart of this task: the human post-editor or the artificial intelligence system?” said Oliver. “We’re convinced that the leading role is played by humans, and that the AI system must be at their service, helping them to become more productive whilst ensuring the final quality of the product.”
According to the researchers, the quality of machine translation has a direct impact on translators’ work. Greater effort entails more time and difficulty in post-editing and this has two clear consequences: it increases the risk of the end result being of poorer quality because the translator in question is unable to catch all the errors and it also increases the time the translator spends on post-editing, which is a waste of money.
“Quality in machine translation is essential for there to be a proper post-editing process,” said Oliver.
Studies like this one by the two UOC researchers also have an important indirect impact: they improve our understanding of machine translation tools, and thereby democratize access to them and ensure that their use does not affect the working conditions of human translators.
“It’s extremely important that our understanding of these technologies and access to these tools is not restricted to just a few specialists and a limited number of companies,” concluded Álvarez-Vidal. “Universities in general, and the UOC in particular, are making great efforts to include an understanding of these technologies in their courses, on both bachelor’s and master’s degree programs.”
More information:
Sergi Alvarez-Vidal et al, Assessing MT with measures of PE effort, Ampersand (2023). DOI: 10.1016/j.amper.2023.100125
Open University of Catalonia
Citation:
Strengthening the partnership between humans and AI: The case of translators (2024, March 13)
retrieved 13 March 2024
from https://techxplore.com/news/2024-03-partnership-humans-ai-case.html
This document is subject to copyright. Apart from any fair dealing for the purpose of private study or research, no
part may be reproduced without the written permission. The content is provided for information purposes only.