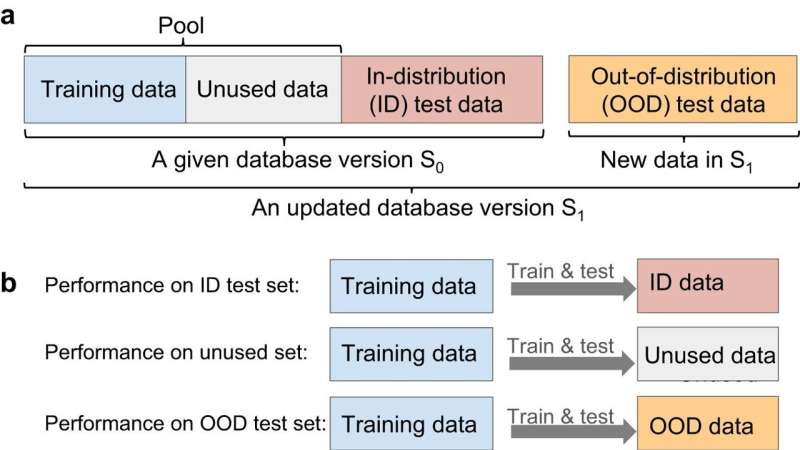
From ChatGPT to DALL-E, deep learning artificial intelligence (AI) algorithms are being applied to an ever-growing range of fields. A new study from University of Toronto Engineering researchers, published in Nature Communications, suggests that one of the fundamental assumptions of deep learning models—that they require enormous amounts of training data—may not be as solid as once thought.
Professor Jason Hattrick-Simpers and his team are focused on the design of next-generation materials, from catalysts that convert captured carbon into fuels to non-stick surfaces that keep airplane wings ice-free.
One of the challenges in the field is the enormous potential search space. For example, the Open Catalyst Project contains more than 200 million data points for potential catalyst materials, all of which still cover only a tiny portion of the vast chemical space that may, for example, hide the right catalyst to help us address climate change.
“AI models can help us efficiently search this space and narrow our choices down to those families of materials that will be most promising,” says Hattrick-Simpers.
“Traditionally, a significant amount of data is considered necessary to train accurate AI models. But a dataset like the one from the Open Catalyst Project is so large that you need very powerful supercomputers to be able to tackle it. So, there’s a question of equity; we need to find a way to identify smaller datasets that folks without access to huge amounts of computing power can train their models on.”
But this leads to a second challenge: Many of the smaller materials datasets currently available have been developed for a specific domain—for example, improving the performance of battery electrodes.
This means that they tend to cluster around a few chemical compositions similar to those already in use today and may be missing possibilities that could be more promising, but less intuitively obvious.
“Imagine if you wanted to build a model to predict students’ final grades based on previous test scores,” says Dr. Kangming Li, a postdoctoral fellow in Hattrick-Simpers’ lab. “If you trained it only on students from Canada, it might do perfectly well in that context, but it might fail to accurately predict grades for students from France or Japan. That’s the situation we are up against in the world of materials.”
One possible solution to address the above challenges is to identify subsets of data from within very large datasets that are easier to process, but which nevertheless retain the full range of information and diversity present in the original.
To better understand how the qualities of datasets affect the models they are used to train, Li designed methods to identify high-quality subsets of data from previously published materials datasets, such as JARVIS, The Materials Project, and the Open Quantum Materials Database (OQMD). Together, these databases contain information on more than a million different materials.
Li built a computer model that predicted material properties and trained it in two ways: One used the original dataset, but the other used a subset of that same data that was approximately 95% smaller.
“What we found was that when trying to predict the properties of a material that was contained within the domain of the dataset, the model that had been trained on only 5% of the data performed about the same as the one that had been trained on all the data,” says Li. “Conversely, when trying to predict the properties of a material that was outside the domain of the dataset, both of them did similarly poorly.”
Li says that the findings suggest a way of measuring the amount of redundancy in a given dataset: if more data does not improve model performance, it could be an indicator that those additional data are redundant and do not provide new information for the models to learn.
“Our results also reveal a concerning degree of redundancy hidden within these highly sought-after large datasets,” says Li.
The study also underlines what AI experts from many fields are finding to be true: that even models trained on relatively small datasets can perform well if the data is of high enough quality.
“All this grew out of the fact that in terms of using AI to speed up materials discovery, we’re just getting started,” says Hattrick-Simpers.
“What it suggests is that as we go forward, we need to be really thoughtful about how we build our datasets. That’s true whether it’s done from the top down, as in selecting a subset of data from a much larger dataset, or from the bottom up, as in sampling new materials to include.
“We need to pay attention to the information richness, rather than just gathering as much data as we can.”
More information:
Kangming Li et al, Exploiting redundancy in large materials datasets for efficient machine learning with less data, Nature Communications (2023). DOI: 10.1038/s41467-023-42992-y
University of Toronto
Citation:
New study finds bigger datasets might not always be better for AI models (2023, November 13)
retrieved 13 November 2023
from https://techxplore.com/news/2023-11-bigger-datasets-ai.html
This document is subject to copyright. Apart from any fair dealing for the purpose of private study or research, no
part may be reproduced without the written permission. The content is provided for information purposes only.