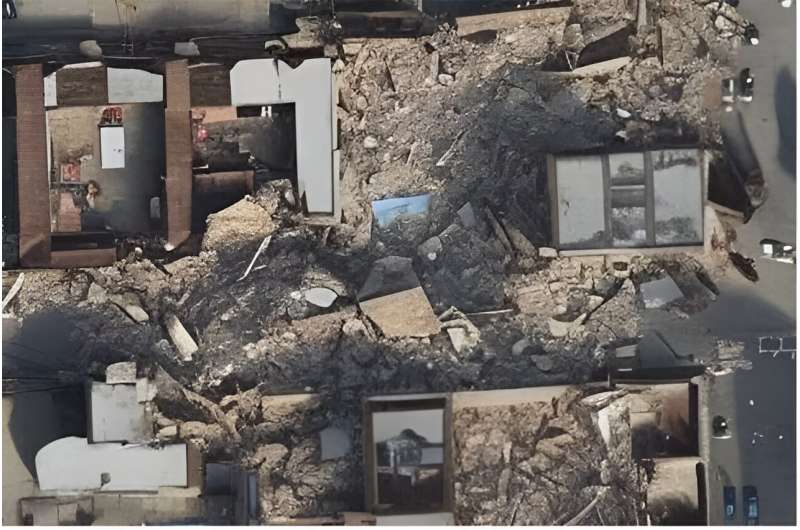
Most people interact with maps regularly, for example, when they’re trying to get from point A to point B, track the weather or plan a trip. But beyond those daily activities, maps are also increasingly being combined with artificial intelligence to create powerful tools for urban modeling, navigation systems, natural hazard forecasting and response, climate change monitoring, virtual habitat modeling and other kinds of surveillance.
“Maps are a fundamental product in our life,” said Aayush Dhakal, a graduate student in the McKelvey School of Engineering at Washington University in St. Louis. “They allow us to learn patterns and see distributions across geospatial area.”
Dhakal and Srikumar Sastry, also a McKelvey Engineering graduate student, are working with Nathan Jacobs, professor of computer science & engineering, to develop models that use satellite imagery to support these endeavors. Dhakal’s project, Sat2Cap, allows users to create maps from free-form textual descriptions. Sastry developed GeoSynth, a model for synthesizing satellite images based on a given textual prompt or geographic location.
Dhakal and Sastry presented their work at this year’s June 17 EarthVision workshop in Seattle in conjunction with the Computer Vision and Pattern Recognition Conference (CVPR 2024). EarthVision aims to advance machine learning-based analysis of remote sensing data with particular attention to urgent challenges and applications, such as monitoring natural hazards, urban growth, deforestation and climate change.
Mapping text from satellite images
Creating a map can be a time-consuming process. A would-be cartographer must collect all the relevant data for the region of interest, then carefully plot it to produce an accurate map. Dhakal developed Sat2Cap as a solution to this “tedious and not scalable” map-making process. The paper won the Best Paper Award at the workshop and is available on the arXiv preprint server.
“Our model allows us to create maps of any concept that is expressed using text over a large geographic region,” Dhakal said. “We contrastively trained a model that takes as input a satellite image over a location and learns to predict meaningful textual representation for that location.”
The tricky part, Dhakal says, is large-scale data collection. Based on many satellite images—Dhakal used 6 million data points to train Sat2Cap—the model can produce a map showing likely locations for a given text query. For example, say the model has lots of images of the United States. If you give it the text prompt, “amusement parks,” the model will produce a map showing the most likely locations that contain amusement parks across the U.S.
“We describe this process as ‘zero-shot mapping,’ where you can create maps of never-before-seen concepts, as opposed to laborious data collection,” Dhakal said. “People might use this tool to map concepts for which data is not yet collected or available. The ability to interact with our model using ‘natural human language’ also makes it much more friendly and flexible.”
High-resolution satellite image synthesis
Generative artificial intelligence has gotten a lot of hype lately, but just how capable are generative models?
“Generating satellite images is much more difficult than generating single-subject images like dogs and cats,” Sastry said. With GeoSynth, he set out to see how well generative models could perform when trained on geographic location data. The paper is also available on the arXiv preprint server.
“The key obstacle was to condition the diffusion model on geographic location to learn a region’s high-level geography,” Sastry said. “For example, when told to generate an image from Phoenix, the model should generate a desert-looking image. On the other hand, for Des Moines, the model should generate more greenish and farm-like images.”
The resulting GeoSynth model displays zero-shot satellite image generation capability. Given a text prompt or geographic location, the model can produce satellite images ranging from flooded cities to island resorts, scenes of post-earthquake destruction to arctic civilizations. Notably, these images are distinct from the kinds of images seen in the training dataset.
“Imagine a scenario where you describe a scene and a layout and suddenly a realistic satellite image blooms into existence,” Sastry said. “GeoSynth can do that. The model could be used for planning cities, augmenting existing remote sensing datasets or as a generative tool, similar to DALLE-3 or Midjourney.”
More information:
Aayush Dhakal et al, Sat2Cap: Mapping Fine-Grained Textual Descriptions from Satellite Images, arXiv (2023). DOI: 10.48550/arxiv.2307.15904
Srikumar Sastry et al, GeoSynth: Contextually-Aware High-Resolution Satellite Image Synthesis, arXiv (2024). DOI: 10.48550/arxiv.2404.06637
arXiv
Washington University in St. Louis
Citation:
Artificial intelligence meets cartography: Mapping tools can create satellite images from text prompts (2024, July 18)
retrieved 18 July 2024
from https://techxplore.com/news/2024-07-artificial-intelligence-cartography-tools-satellite.html
This document is subject to copyright. Apart from any fair dealing for the purpose of private study or research, no
part may be reproduced without the written permission. The content is provided for information purposes only.