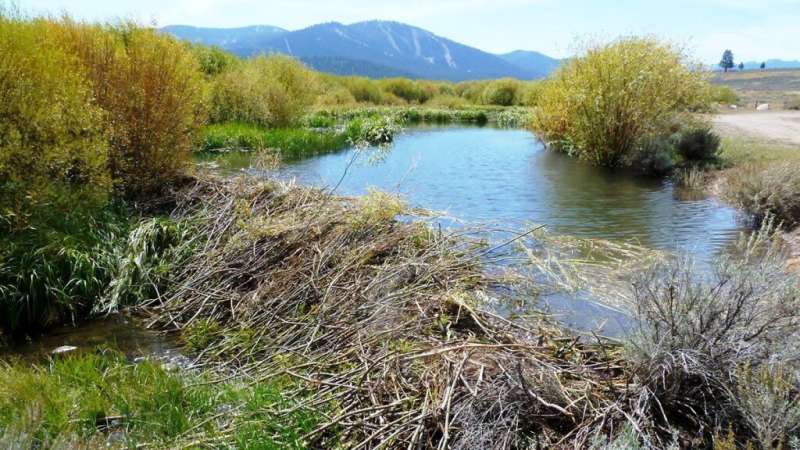
North American beavers transform ecosystems with their engineering prowess. By ponding water, excavating channels, and foraging nearby vegetation, they drastically alter landscapes across a variety of environments, from tundra to deserts.
Two centuries of fur trading starting in the 17th century decimated the thick-coated builders, but today, beaver populations are rebounding gradually. That’s good news for many ecosystems because beaver construction creates valuable habitats for endangered species, traps carbon, and improves water availability in dry places.
Despite these ecologic implications, large-scale mapping of beaver habitats has been missing from scientific research. Most mapped dams are identified manually, which takes a lot of time and effort.
To accelerate beaver dam identification, Emily Fairfax and colleagues applied machine learning to scour high-resolution geospatial imagery for likely dam complexes across landscape- and regional-scale areas. The researchers developed the Earth Engine Automated Geospatial Element(s) Recognition (EEAGER) model, which uses a neural network trained on thousands of known locations of beaver dams in aerial and satellite images. Their study is published in the Journal of Geophysical Research: Biogeosciences.
In this study, the team trained the model to identify dams in the western United States, then tested it outside the training areas to see whether it correctly spotted other dams in imagery. Overall, EEAGER was 98.5% accurate in characterizing whether sites in imaged landscapes did or did not have dams. Both the model’s recall (the percentage of known dams correctly identified by the model) and precision (the percentage of model-predicted dams that were, indeed, dams), at 63% and 26%, respectively, could be improved with additional training in different regions where beavers build, the authors note.
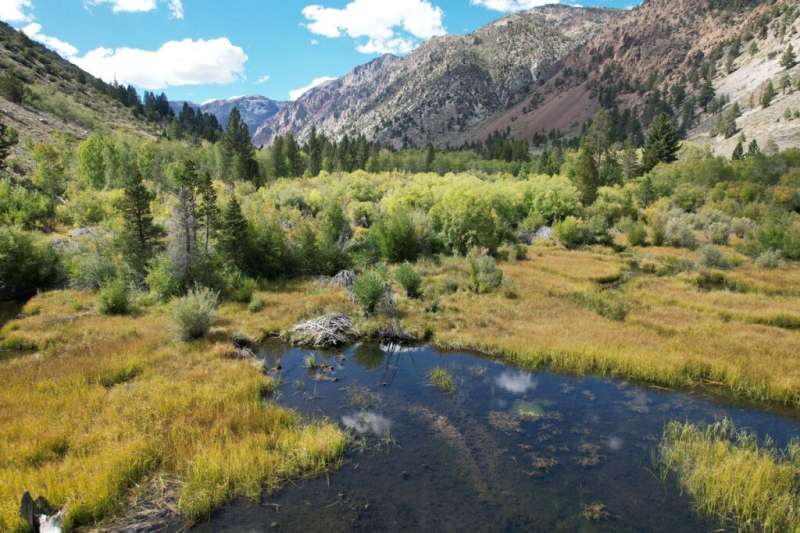
Still, the relatively high recall is a good sign, they say, suggesting the model can detect a large proportion of actual dams. They also pointed out that false-positive dam identifications can be easily removed from the catalog manually and that many false positives were close to actual beaver dams.
This work can help track beaver populations and health as well as ecosystem changes and beaver-based river restoration, the researchers note, and the methodology could be applied to monitor other complex landforms across large regions.
More information:
Emily Fairfax et al, EEAGER: A Neural Network Model for Finding Beaver Complexes in Satellite and Aerial Imagery, Journal of Geophysical Research: Biogeosciences (2023). DOI: 10.1029/2022JG007196
This story is republished courtesy of Eos, hosted by the American Geophysical Union. Read the original story here.
Citation:
Mapping beaver dams with machine learning (2023, June 16)
retrieved 16 June 2023
from https://phys.org/news/2023-06-beaver-machine.html
This document is subject to copyright. Apart from any fair dealing for the purpose of private study or research, no
part may be reproduced without the written permission. The content is provided for information purposes only.