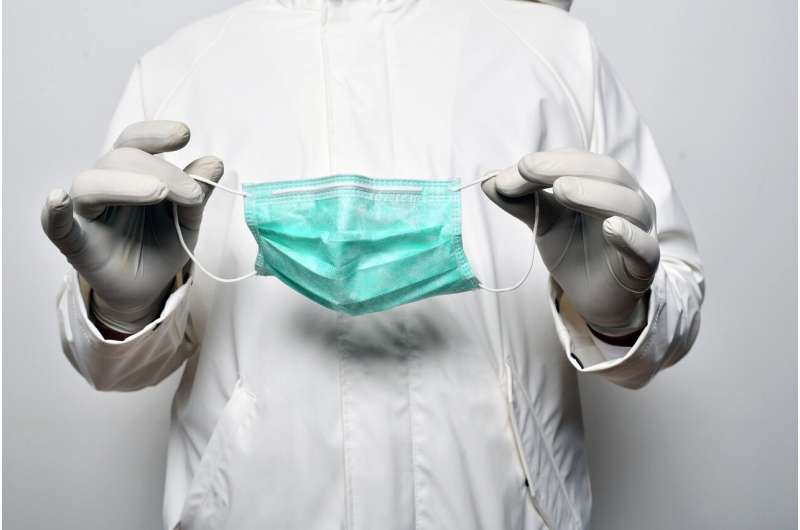
Face mask wearing detection is an important technical approach to improve public health safety and real-time monitoring efficiency. However, under extreme lighting or weather conditions, it is difficult to achieve ideal results with existing object detection or face detection algorithms.
To solve this problem, a research team led by Mingqiang Guo, proposed an end-to-end joint learning optimized detection framework with layer decomposition enhancement and adaptive multi-scale feature fusion. The team published their new research in Frontiers of Computer Science.
On the one hand, the LLE module recovers brightening feature information through layer decomposition and component adjustment constraints. The image decomposition is implemented based on Retinex rule, resulting in the illumination component and the reflectance component. Then the features on the spaces of the two components are enhanced by the designed mathematical constraint terms, respectively.
On the other hand, the object detector achieves adaptive multi-scale feature fusion through the spatially coordinated attention mechanism and CW-FPN module.
In order to provide a more thorough evaluation of the comprehensive performance of the model, they launched a series of comparative experimental analyses on two public benchmark datasets, DARK FACE and PWMFD. Ultimately the proposed model achieves exciting results in terms of both detection capability and efficiency.
More information:
Mingqiang Guo et al, CW-YOLO: joint learning for mask wearing detection in low-light conditions, Frontiers of Computer Science (2023). DOI: 10.1007/s11704-023-3351-y
Provided by
Frontiers Journals
Citation:
Joint learning for mask wearing detection in low-light conditions (2024, February 5)
retrieved 5 February 2024
from https://techxplore.com/news/2024-02-joint-mask-conditions.html
This document is subject to copyright. Apart from any fair dealing for the purpose of private study or research, no
part may be reproduced without the written permission. The content is provided for information purposes only.