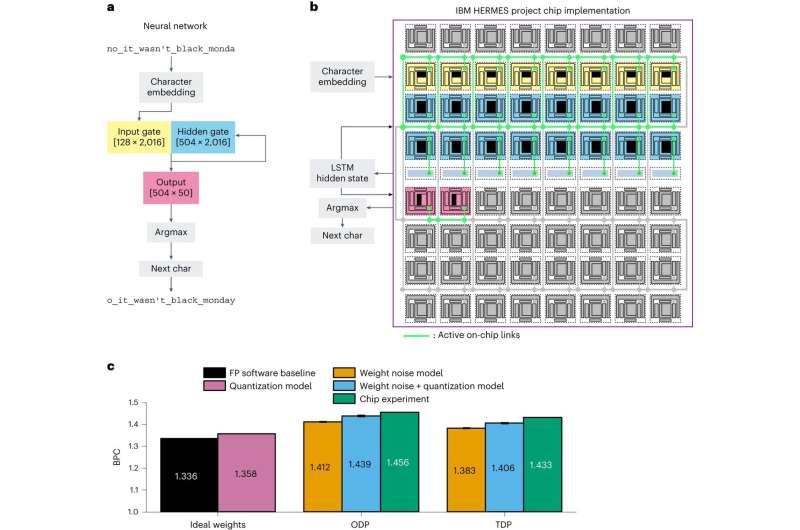
Deep neural networks are generating much of the exciting progress stemming from generative AI. But their architecture relies on a configuration that is a virtual speedbump, ensuring the maximal efficiency can not be obtained.
Constructed with separate units for memory and processing, neural networks face heavy demands on system resources for communications between the two components that results in slower speeds and reduced efficiency.
IBM Research came up with a better idea by turning to the perfect model for its inspiration for a more efficient digital brain: the human brain.
In a paper, “A 64-core mixed-signal in-memory compute chip based on phase-change memory for deep neural network inference,” published in Nature Electronics Aug. 10, IBM researchers said they applied a new approach for a state-of-the-art mixed-signal AI chip that promises to improve efficiency and incur less battery drain in AI projects.
“The human brain is able to achieve remarkable performance while consuming little power,” said one of the co-authors of the study, Thanos Vasilopoulos, of IBM’s research lab in Zurich, Switzerland.
Acting in similar fashion to the way synapses interact with one another in the brain, IBM’s mixed-signal chip features 64 analog in-memory cores with each one hosting an array of synaptic cell units. Converters ensure smooth transitions between analog and digital states.
The chips, according to IBM, achieved a 92.81% accuracy rate on the CIFAR-10 dataset, a widely used collection of images used in training for machine learning.
“We demonstrate near-software-equivalent inference accuracy with ResNet and long short-term memory networks,” Vasilopoulos said. ResNet, short for residual neural network, is a deep learning model that allows training on thousands of layers of a neural network without hindering performance.
“To achieve end-to-end improvements in latency and energy consumption, AIMC must be combined with on-chip digital operations and on-chip communication,” Vasilopoulos stated. “Here we report a multicore AIMC chip designed and fabricated in 14 nm complementary metal–oxide–semiconductor technology with backend-integrated phase-change memory.”
With such improved performance, Vasilopoulos said, “large and more complex workloads could be executed in low power or battery-constrained environments.” This would include cellphones, cars and cameras.
“Additionally, cloud providers will be able to use these chips to reduce energy costs and their carbon footprint,” he said.
IBM said that with future improvements in digital circuitry allowing layer-to-layer activation transfers and intermediate activation storage in local memory will allow the execution of fully pipelined end-to-end inference workloads on these chips.
On his personal blog discussing the latest IBM achievement, Vasilopoulos said, “With this work, many components needed to fully realize the promise of Analog-AI, for performant and power efficient AI, have been silicon-validated.”
He offered a technical overview of the chip in a separate article titled, “Analog in-memory computing coming of age,” published in Electrical and Electronic Engineering Aug. 10.
Referring to the chip as “the first of its kind,” he described it as “a fully integrated mixed-signal in-memory compute chip based on back-end integrated phase-change memory (PCM) in a 14-nm complementary metal-oxide-semiconductor (CMOS) process.”
Further defining the project, he said, “The chip comprises 64 AIMC cores, each with a memory array of 256×256 unit cells. The unit cells are constructed with four PCM devices for a total of over 16M devices. In addition to the analog memory array, each core contains a light digital processing unit performing activation functions, accumulations, and scaling operations.”
More information:
Manuel Le Gallo et al, A 64-core mixed-signal in-memory compute chip based on phase-change memory for deep neural network inference, Nature Electronics (2023). DOI: 10.1038/s41928-023-01010-1. On arXiv: DOI: 10.48550/arxiv.2212.02872
Technical overview: Analog in-memory computing coming of age
© 2023 Science X Network
Citation:
IBM reports analog AI chip patterned after human brain (2023, August 22)
retrieved 22 August 2023
from https://techxplore.com/news/2023-08-ibm-analog-ai-chip-patterned.html
This document is subject to copyright. Apart from any fair dealing for the purpose of private study or research, no
part may be reproduced without the written permission. The content is provided for information purposes only.