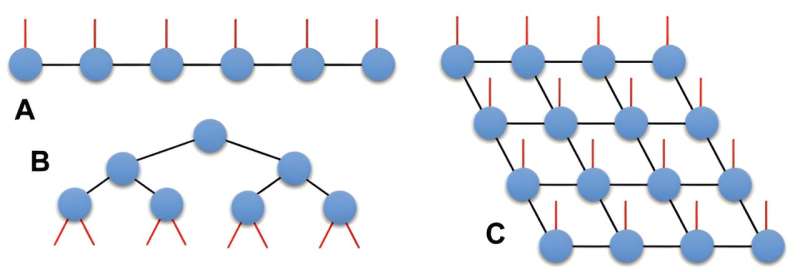
Deep machine learning has achieved remarkable success in various fields of artificial intelligence, but achieving both high interpretability and high efficiency simultaneously remains a critical challenge. Shi-Ju Ran of Capital Normal University and Gang Su of the University of the Chinese Academy of Sciences have reviewed an innovative approach based on tensor networks, drawing inspiration from quantum mechanics. This approach offers a promising solution to the long-standing challenge of reconciling interpretability and efficiency in deep machine learning.
The review was published Nov. 17 in Intelligent Computing.
Deep machine learning models, especially neural network models, are often considered “black boxes” because their decision-making processes are complex and difficult to explain. According to the authors, “Neural networks, the most powerful machine learning models nowadays, have evolved through decades of delicate designs and optimizations, backed by significant human and capital investments. A typical example showcasing their power is the generative pretraining transformers (GPTs). However, due to the lack of interpretability, even the GPTs suffer from severe problems such as robustness and the protection of privacy.”
The lack of interpretability of these models can lead to a lack of trust in their predictions and decisions, limiting their applications in important areas.
Tensor networks, based on quantum information and many-body physics, provide a “white-box” approach to machine learning. The authors state, “Tensor networks play a crucial role in bridging quantum concepts, theories, and methods with machine learning and in efficiently implementing tensor network-based machine learning.”
Serving as a mathematical framework, they efficiently represent high-dimensional data or functions, using tensor products to compactly structure multi-dimensional information.
A novel, intrinsically interpretable tensor network-based machine learning framework has emerged. This innovative approach efficiently constructs a probabilistic machine learning model from quantum states represented and simulated by tensor networks. Remarkably, the interpretability of this framework is not only comparable to classical probabilistic machine learning but may even surpass it. This quantum-inspired machine learning scheme introduces fresh perspectives by integrating physical concepts such as entanglement entropy and quantum correlations into machine learning investigations, thereby significantly enhancing interpretability.
To enhance efficiency, the quantum-inspired tensor network-based machine learning framework must be combined with quantum computational methods and techniques. Tensor networks play a pivotal role in representing quantum operations, serving as mathematical models for intricate processes in quantum mechanics. This innovative approach leverages tensor networks as mathematical representations of quantum circuit models, similar to classical logical circuits. Their efficient handling of quantum gates, executable on various quantum platforms, is key to the success of this approach.
Tensor networks, known for their efficiency in classical computation, offer stable access to qubits, surpassing in several cases the capabilities of quantum computers in the noisy intermediate-scale quantum area. The integration of tensor networks in quantum-inspired machine learning not only addresses the challenges associated with high-dimensional spaces but also enhances the efficiency of machine learning schemes on quantum platforms.
Furthermore, tensor networks find applications in machine learning beyond quantum probabilistic interpretations. They efficiently represent and simulate partition functions of classical stochastic systems, contributing to the enhancement of regular neural networks and the creation of novel machine learning models. Tensor networks play a crucial role in simplifying tasks such as dimensionality reduction, feature extraction and the implementation of support vector machines, showcasing their versatility.
In conclusion, tensor networks offer a breakthrough in addressing the efficiency-interpretability challenge in artificial intelligence, especially in quantum-inspired machine learning.
The authors observe, “Based on previous research, we believe that with more time and investments, tensor networks will eventually achieve equal or higher accuracies, along with improved interpretability, compared to neural networks. We would see tensor networks as a fundamental mathematical tool for studying artificial intelligence, especially when we are equipped with quantum computing hardware.”
Their unique ability to blend quantum theories for interpretability and quantum methods for efficiency positions tensor networks as a key tool in navigating the complexities of emergent artificial intelligence.
More information:
Shi-Ju Ran et al, Tensor Networks for Interpretable and Efficient Quantum-Inspired Machine Learning, Intelligent Computing (2023). DOI: 10.34133/icomputing.0061
Provided by
Intelligent Computing
Citation:
Tensor networks: Enhancing interpretability and efficiency in quantum-inspired machine learning (2023, November 27)
retrieved 27 November 2023
from https://techxplore.com/news/2023-11-tensor-networks-efficiency-quantum-inspired-machine.html
This document is subject to copyright. Apart from any fair dealing for the purpose of private study or research, no
part may be reproduced without the written permission. The content is provided for information purposes only.