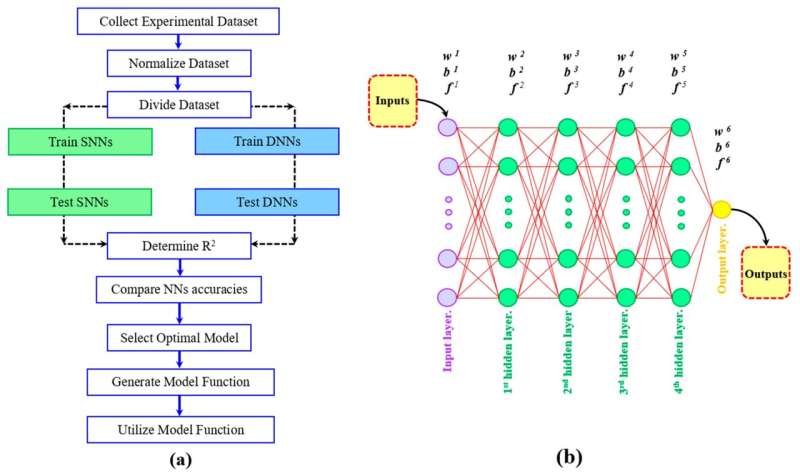
Metal matrix composites are a modern alternative to steel. They are molecular-reinforced materials, which consist of a metal reinforcement matrix and filler. Such composites can be further strengthened, for example, using titanium monobromide or titanium carbide.
But even such strong materials are not immune to destruction, so they are additionally processed by shot blasting (or shot peening): a powerful gas jet with small particles makes it stronger. To select the optimal parameters for shot peening, an extensive experimental base is needed. However conducting such experiments is expensive and time-consuming, and existing simulators do not give plausible results. A RUDN University professor and colleagues from Italy, Canada, and Turkey have trained a deep neural network for this.
The research is published in the journal Materials.
“Most failure in metallic materials starts at the surface. This also happens in critical parts of industrial production. Therefore, shot peening can play a decisive role and improve the mechanical properties of the surface—hardness, resistance to corrosion and wear. Testing in this area is labor-intensive and expensive. Simulation programs contain many errors, which can lead to catastrophic damage,” said Kazem Reza Kashyzadeh, professor of the RUDN Department of Transport.
To train the neural network, the researchers used experimental data from shot-peened titanium composites with different contents of reinforcers. The researchers took the content of amplifiers—monobromide and titanium carbide—and the intensity of processing as input data. At the output, the neural network described the hardness and residual stresses throughout the entire depth of the material.
The accuracy of the constructed neural network turned out to be almost perfect. The material hardness was predicted with 99.4% accuracy, and the residual stress with 98.8% accuracy. Previous results obtained with a shallow neural network were about 1% less accurate. A neural network helps to clarify how the intensity of processing will affect the result. For example, the most pronounced residual stress appears at a depth of up to 15 micrometers from the surface at an intensity of 0.25-0.30 on the Almen scale.
“The predictions of the deep neural network were 0.98% more accurate than those of the conventional one. Therefore, deep neural networks can be considered a powerful tool for analyzing hardness and residual stress after shot peening,” said Professor Kashyzadeh.
More information:
Erfan Maleki et al, A Novel Approach for Analyzing the Effects of Almen Intensity on the Residual Stress and Hardness of Shot-Peened (TiB + TiC)/Ti–6Al–4V Composite: Deep Learning, Materials (2023). DOI: 10.3390/ma16134693
RUDN University
Citation:
AI predicts the strength of a composite reinforced with titanium carbide and bromide after processing (2024, January 10)
retrieved 10 January 2024
from https://techxplore.com/news/2024-01-ai-strength-composite-titanium-carbide.html
This document is subject to copyright. Apart from any fair dealing for the purpose of private study or research, no
part may be reproduced without the written permission. The content is provided for information purposes only.