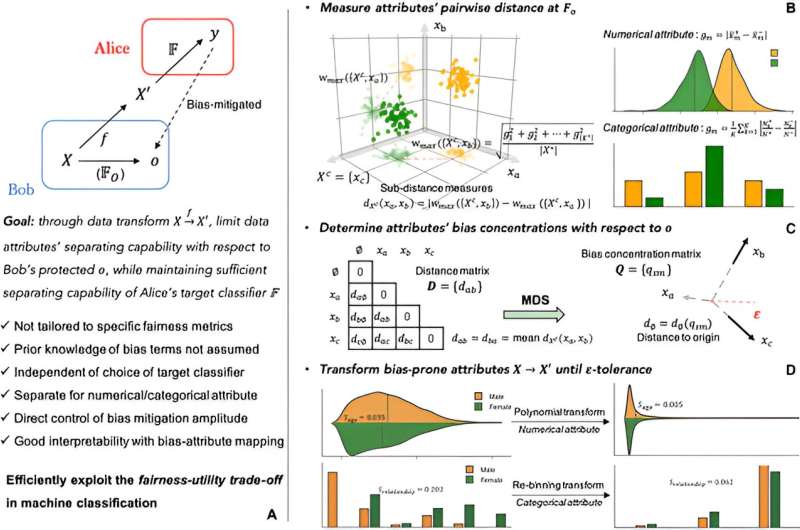
We use computers to help us make (hopefully) unbiased decisions. The problem is that machine-learning algorithms do not always make fair classifications if human bias is embedded in the data used to train them—which is often the case in practice.
To ease this “garbage in, garbage out” situation, a research team presented a flexible framework for mitigating bias in machine classification. Their research was published in Intelligent Computing.
Existing attempts to mitigate classification bias, according to the team, are often held back by their reliance on specific metrics of fairness and predetermined bias terms. The team’s framework avoids these two types of reliance; their bias mitigation can be evaluated under different fairness metrics, and they infer specific bias terms from the data.
The team evaluated the framework on seven datasets across 21 machine classifiers. Across the experiments, bias in classification outcomes is substantially reduced, with classification accuracy largely preserved—working desirably under the fairness-utility trade-off.
The framework shares the setup of the adversarial debiasing method, considering a propose-review scenario between Alice, e.g., the enterprise, and Bob, e.g., the regulator. Alice sends a proposal to Bob for using his data to develop a target classifier, say, a college matching system.
Bob reviews the proposal and aims to make sure that Alice’s classification does not demonstrate substantial bias along a sensitive dimension that he aims to protect, say, students’ middle-school transfer experience. The goal is to build a classifier that has minimal discrimination along the protected dimension(s) with only a small performance sacrifice in the target classification.
Bias mitigation is achieved by identifying data attributes that are prone to introducing bias and then applying effective data transforms on records under these attributes.
This involves assessing the contribution of attributes to data separation, computing the distances between attributes, and establishing with these distances a bias-attribute mapping in the constructed bias hyperspace. With this mapping, bias terms are inferred, bias-prone attributes are recognized, and their bias concentrations are measured.
However, the workflow may encounter difficulties when applied to large datasets due to limitations in scalability, among other factors.
In future research, the team is interested in extending the framework to directly strike a balance between classification fairness and accuracy, considering the potential conflict between the public and private sectors. From a broader standpoint, incorporating behavioral features into classification bias mitigation and analyzing practical setups in the application of such frameworks is an important direction.
More information:
Zhoufei Tang et al, Metric-Independent Mitigation of Unpredefined Bias in Machine Classification, Intelligent Computing (2024). DOI: 10.34133/icomputing.0083
Intelligent Computing
Citation:
New mitigation framework reduces bias in classification outcomes (2024, April 23)
retrieved 1 May 2024
from https://techxplore.com/news/2024-04-mitigation-framework-bias-classification-outcomes.html
This document is subject to copyright. Apart from any fair dealing for the purpose of private study or research, no
part may be reproduced without the written permission. The content is provided for information purposes only.