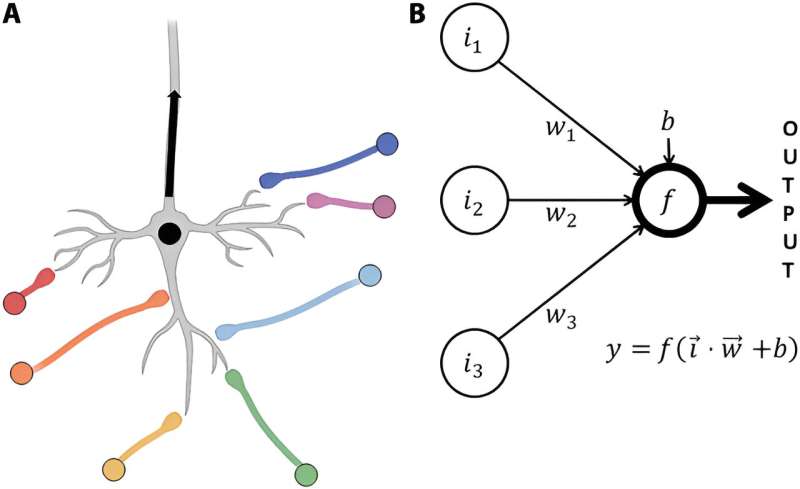
Researchers have made significant progress in the development of artificial neural networks using tiny silicon devices called microresonators, paving the way for faster and more energy-efficient artificial intelligence systems. These networks mimic the computing capabilities of the human brain, breaking away from traditional digital computer architectures and leveraging the speed, low power dissipation and multi-wavelength capabilities of photonics.
A review article describing the implementations of neural networks using silicon microresonators was published in Intelligent Computing.
Silicon microresonators are tiny structures that trap and confine light. Silicon microring resonators are loop-like microresonators that guide light in a circular path. In optical systems, they can trap light and change its intensity, allowing precise control of the properties of the light, such as its frequency, phase and amplitude. These resonators could be applied in optical communications and sensing.
One useful feature of microring resonators is their ability to store high field intensity, which enhances the light-matter interaction. This makes the nonlinear response available at relatively low power, allowing them to mimic biological neurons. When the energy level of the light is low, microring resonators behave predictably, responding to the input light linearly. This means that if the light input increases, the output light increases proportionally.
However, at higher energy levels, microring resonators enter a nonlinear regime, meaning the output or the behavior of the light does not change in direct proportion to the input. This is because the light itself starts to affect the properties of the material, such as its refractive index and its ability to absorb light.
In biological neurons, input signals are received, processed and transformed into output signals. This transformation is often nonlinear because a neuron might suddenly fire when the input reaches a certain threshold. Because the way that microring resonators change the behavior of light is similar to how our brain cells work, they can be used to emulate neural activity in artificial neural networks.
Another useful feature of microring resonators is their sensitivity to wavelength, which allows them to serve as weight banks. In artificial neural networks, weights are parameters that determine the strength of the connections between neurons, influencing the flow of information and the ability of the network to learn complex patterns. Silicon microring resonators can act as weight banks in photonic neural networks.
They work by controlling how much of an incoming light signal gets through, depending on its wavelength. This control allows microring resonators to adjust the “weight” of each incoming light signal and is crucial for learning and adapting in neural networks. The range of these weights depends on how well the microring resonators can block light, which is determined by their design and the materials they are made from.
More information:
Stefano Biasi et al, Photonic Neural Networks Based on Integrated Silicon Microresonators, Intelligent Computing (2023). DOI: 10.34133/icomputing.0067
Intelligent Computing
Citation:
Exploring the use of silicon microresonators for artificial neural networks (2024, February 22)
retrieved 22 February 2024
from https://techxplore.com/news/2024-02-exploring-silicon-microresonators-artificial-neural.html
This document is subject to copyright. Apart from any fair dealing for the purpose of private study or research, no
part may be reproduced without the written permission. The content is provided for information purposes only.