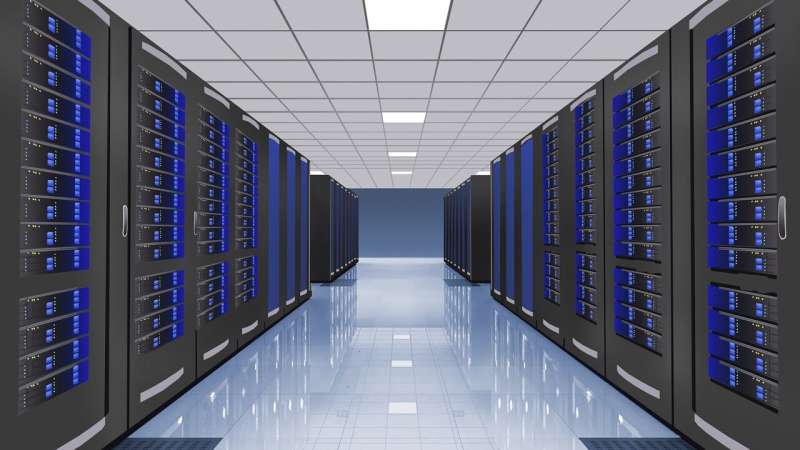
Some recent mergers, acquisitions and investments in the business world have highlighted the strategic value of data to companies. These businesses are not just buying assets or market share—they are also acquiring or investing in large, complementary datasets. This process is known in the business world as horizontal integration.
This integration can drive innovation and provide competitive advantages. It can also open up new revenue streams. Some examples include Microsoft’s acquisitions of LinkedIn and GitHub as well as Amazon’s acquisitions of WholeFoods and the Washington Post. Then there has been Discovery Communications’ merger with Warner Brothers, IBM’s investment in Hugging Face and Google’s investment in Anthropic.
As the last two examples illustrate, data is extremely important for AI companies. It’s vital for “training,” or improving, AI systems. Training AI systems on large, new, varied data sets allows companies to develop more advanced, more powerful AI systems.
But against the background of this scramble, there is also a growing consensus that some form of regulation is needed to address the ethical, safety and fairness concerns associated with AI.
But regulating AI presents a unique set of challenges. This is mainly due to its foundation on intangible elements such as software and algorithms. These elements can be easily modified, replicated and distributed across borders with few physical traces. This helps them evade traditional regulatory mechanisms that rely on controlling physical goods or specific locations.
Yet a promising approach to regulating AI is one that would focus on controlling access to the very data that is the lifeblood of AI development. Since data is behind the rise of horizontal integration as well as fueling the growth and sophistication of AI systems, its concentration in the hands of a few entities can lead to monopolistic dominance. In short, it gives too much power to too few companies.
Antitrust model
To mitigate this, regulatory frameworks could be designed that resemble existing antitrust laws—but focused around data aggregation. They would help ensure a diverse and competitive landscape in the access to data. By preventing any single company from amassing an overwhelming data advantage, these regulations would aim to foster a more balanced field. Innovation must be allowed to thrive without being stifled by monopolistic control.
To properly achieve this outcome, we suggest that regulators need to look at limiting horizontal integration. As AI technologies continue to evolve and the demand for diverse and extensive datasets grows, companies will increasingly be motivated to pursue horizontal integration.
This trend towards integration not only consolidates data assets but also potentially reduces competition, as fewer companies come to control larger shares of valuable data. Therefore, regulatory scrutiny of such mergers and acquisitions becomes essential to ensure a competitive landscape where data does not become excessively concentrated in a few hands.
It’s important to note that the trend towards horizontal integration is already moderated to some extent by regulatory and ethical considerations, particularly around data privacy and existing antitrust laws. These considerations play a critical role in shaping the extent and nature of integration.
The benefits of more data
When organizations integrate horizontally, they access a more comprehensive pool of data, filling gaps present in individual datasets. This amalgamation not only improves the reliability and accuracy of data but also broadens the perspective, offering deeper insights that are crucial for making informed decisions.
For instance, in merging customer demographic data with purchase history, companies can gain a more nuanced understanding of consumer behavior. This is invaluable in today’s customer-centric market landscape.
Horizontal integration for AI aligns helps modern companies with their operational efficiency. Companies with similar markets or customer bases can optimize their processes based on richer, more comprehensive data insights.
This leads to improved efficiency in data collection and analysis. This is because making use of existing complementary datasets is more efficient and cost-effective than generating new data from scratch. Companies that successfully use combined datasets can better understand and predict customer needs and market trends. This advantage is especially important in industries where innovation and adaptability are key to survival and growth.
A balancing act
Despite the benefits for companies, the potential harm to market competition and consumer welfare from data consolidation necessitates a response. Centralizing extensive datasets under dominant entities can potentially marginalize smaller competitors and stifle market diversity.
It also poses privacy concerns and amplifies the risk of market manipulation, diminishing consumer choice and impeding innovation. The potential benefits of data consolidation for customers include enhanced product offerings and personalized services. It is crucial that regulatory frameworks adopt a “rule of reason” approach. They would diligently scrutinize these activities under merger laws or abuse of dominance laws. This ensures a balanced market ecosystem, mitigates potential harm and safeguards competition and consumer interests.
In conclusion, the argument for horizontal integration in the age of AI is compelling. The synthesis of complementary datasets through such integration offers enhanced data quality, improved AI and machine learning capabilities. It provides operational efficiencies and strategic market advantages.
But we must take a balanced approach, weighing the benefits of integration against the ethical implications and regulatory compliance. The future of business in the AI era will likely be characterized by a continued trend towards strategic integration, shaping the way companies operate and compete.
If left unchecked, horizontal integration will concentrate the power of data in the hands of a few. This which will raise safety concerns and is likely to inhibit competition. But regulation based around antitrust principles—where an organization steps in to prevent companies from behaving in ways that exclude competitors—could help prevent this.
The Conversation
This article is republished from The Conversation under a Creative Commons license. Read the original article.
Citation:
AI companies are merging or collaborating to even out gap in access to vital datasets (2024, January 31)
retrieved 31 January 2024
from https://techxplore.com/news/2024-01-ai-companies-merging-collaborating-gap.html
This document is subject to copyright. Apart from any fair dealing for the purpose of private study or research, no
part may be reproduced without the written permission. The content is provided for information purposes only.