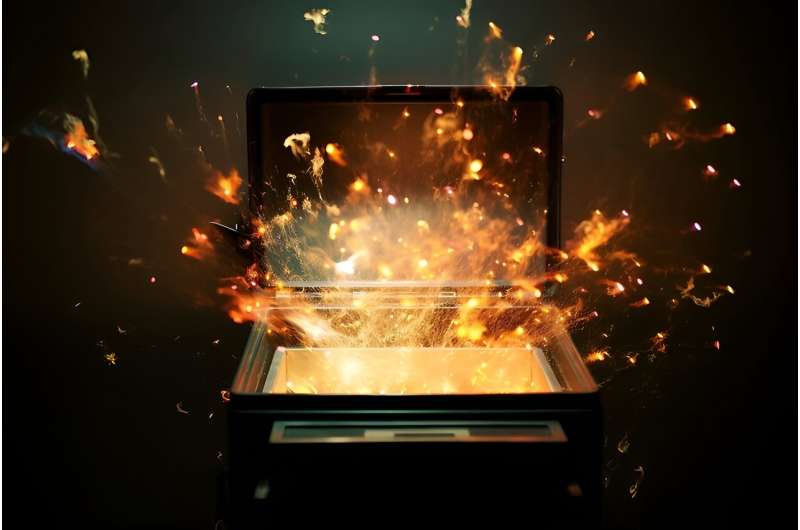
Generative AI, which is currently riding a crest of popular discourse, promises a world where the simple transforms into the complex—where a simple distribution evolves into intricate patterns of images, sounds, or text, rendering the artificial startlingly real.
The realms of imagination no longer remain as mere abstractions, as researchers from MIT’s Computer Science and Artificial Intelligence Laboratory (CSAIL) have brought an innovative AI model to life. Their new technology integrates two seemingly unrelated physical laws that underpin the best-performing generative models to date: diffusion, which typically illustrates the random motion of elements, like heat permeating a room or a gas expanding into space, and Poisson Flow, which draws on the principles governing the activity of electric charges.
This harmonious blend has resulted in superior performance in generating new images, outpacing existing state-of-the-art models. Since its inception, the “Poisson Flow Generative Model ++” (PFGM++) has found potential applications in various fields, from antibody and RNA sequence generation to audio production and graph generation. The work is published on the arXiv preprint server.
The model can generate complex patterns, like creating realistic images or mimicking real-world processes. PFGM++ builds off PFGM, the team’s work from the prior year. PFGM takes inspiration from the means behind the mathematical equation known as the “Poisson” equation, and then applies it to the data the model tries to learn from.
To do this, the team used a clever trick: They added an extra dimension to their model’s “space,” kind of like going from a 2D sketch to a 3D model. This extra dimension gives more room for maneuvering, places the data in a larger context, and helps one approach the data from all directions when generating new samples.
“PFGM++ is an example of the kinds of AI advances that can be driven through interdisciplinary collaborations between physicists and computer scientists,” says Jesse Thaler, theoretical particle physicist in MIT’s Laboratory for Nuclear Science’s Center for Theoretical Physics and director of the National Science Foundation’s AI Institute for Artificial Intelligence and Fundamental Interactions (NSF AI IAIFI), who was not involved in the work.
“In recent years, AI-based generative models have yielded numerous eye-popping results, from photorealistic images to lucid streams of text. Remarkably, some of the most powerful generative models are grounded in time-tested concepts from physics, such as symmetries and thermodynamics,” Thaler explains.
“PFGM++ takes a century-old idea from fundamental physics—that there might be extra dimensions of space-time—and turns it into a powerful and robust tool to generate synthetic but realistic datasets. I’m thrilled to see the myriad of ways ‘physics intelligence’ is transforming the field of artificial intelligence.”
The underlying mechanism of PFGM isn’t as complex as it might sound. The researchers compared the data points to tiny electric charges placed on a flat plane in a dimensionally expanded world. These charges produce an “electric field,” with the charges looking to move upwards along the field lines into an extra dimension and consequently forming a uniform distribution on a vast imaginary hemisphere.
The generation process is like rewinding a videotape: starting with a uniformly distributed set of charges on the hemisphere and tracking their journey back to the flat plane along the electric lines, they align to match the original data distribution. This intriguing process allows the neural model to learn the electric field, and generate new data that mirrors the original.
The PFGM++ model extends the electric field in PFGM to an intricate, higher-dimensional framework. When you keep expanding these dimensions, something unexpected happens—the model starts resembling another important class of models, the diffusion models. This work is all about finding the right balance. The PFGM and diffusion models sit at opposite ends of a spectrum: one is robust but complex to handle, the other simpler but less sturdy.
The PFGM++ model offers a sweet spot, striking a balance between robustness and ease of use. This innovation paves the way for more efficient image and pattern generation, marking a significant step forward in technology. Along with adjustable dimensions, the researchers proposed a new training method that enables more efficient learning of the electric field.
To bring this theory to life, the team resolved a pair of differential equations detailing these charges’ motion within the electric field. They evaluated the performance using the Frechet Inception Distance (FID) score, a widely accepted metric that assesses the quality of images generated by the model in comparison to the real ones. PFGM++ further showcases a higher resistance to errors and robustness toward the step size in the differential equations.
Looking ahead, they aim to refine certain aspects of the model, particularly in systematic ways to identify the “sweet spot” value of D tailored for specific data, architectures, and tasks by analyzing the behavior of estimation errors of neural networks. They also plan to apply the PFGM++ to the modern large-scale text-to-image/text-to-video generation.
“Diffusion models have become a critical driving force behind the revolution in generative AI,” says Yang Song, research scientist at OpenAI. “PFGM++ presents a powerful generalization of diffusion models, allowing users to generate higher-quality images by improving the robustness of image generation against perturbations and learning errors. Furthermore, PFGM++ uncovers a surprising connection between electrostatics and diffusion models, providing new theoretical insights into diffusion model research.”
“Poisson Flow Generative Models do not only rely on an elegant physics-inspired formulation based on electrostatics, but they also offer state-of-the-art generative modeling performance in practice,” says NVIDIA Senior Research Scientist Karsten Kreis, who was not involved in the work.
“They even outperform the popular diffusion models, which currently dominate the literature. This makes them a very powerful generative modeling tool, and I envision their application in diverse areas, ranging from digital content creation to generative drug discovery. More generally, I believe that the exploration of further physics-inspired generative modeling frameworks holds great promise for the future and that Poisson Flow Generative Models are only the beginning,” Kreis adds.
Authors on a paper about this work include three MIT graduate students: Yilun Xu of the Department of Electrical Engineering and Computer Science (EECS) and CSAIL, Ziming Liu of the Department of Physics and the NSF AI IAIFI, and Shangyuan Tong of EECS and CSAIL, as well as Google Senior Research Scientist Yonglong Tian, Ph.D. MIT professors Max Tegmark and Tommi Jaakkola advised the research.
More information:
Yilun Xu et al, PFGM++: Unlocking the Potential of Physics-Inspired Generative Models, arXiv (2023). DOI: 10.48550/arxiv.2302.04265
arXiv
Massachusetts Institute of Technology
This story is republished courtesy of MIT News (web.mit.edu/newsoffice/), a popular site that covers news about MIT research, innovation and teaching.
Citation:
From physics to generative AI: An AI model for advanced pattern generation (2023, September 27)
retrieved 27 September 2023
from https://techxplore.com/news/2023-09-physics-generative-ai-advanced-pattern.html
This document is subject to copyright. Apart from any fair dealing for the purpose of private study or research, no
part may be reproduced without the written permission. The content is provided for information purposes only.